AI-Powered Search: The Missing Piece of Your Drug Development Process
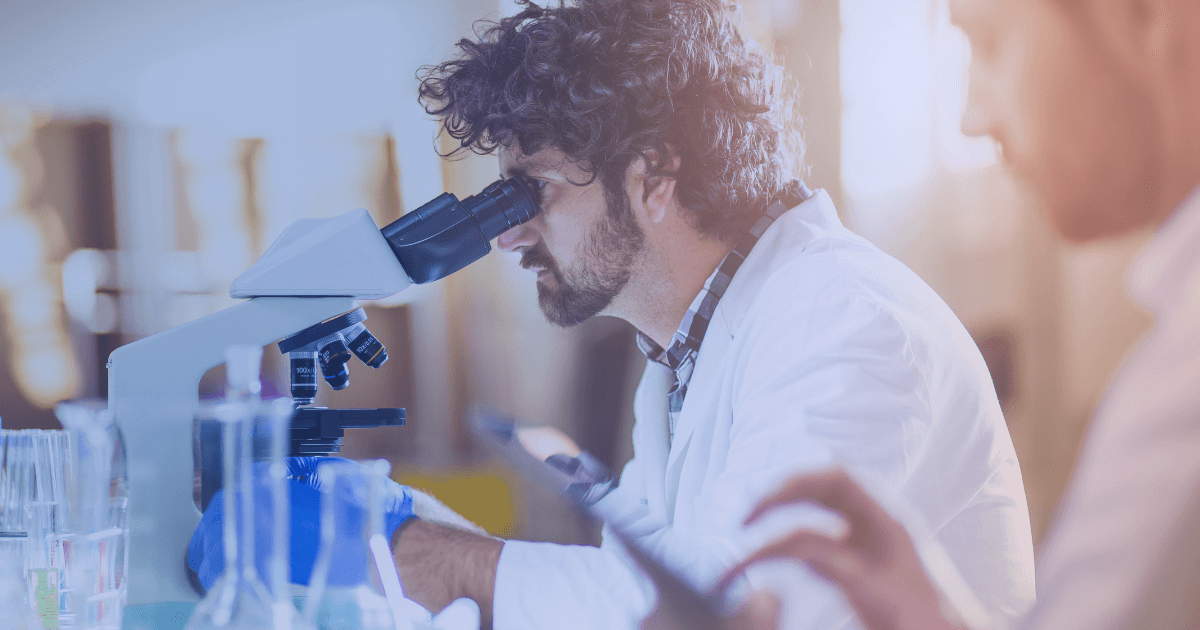
Drug development is a complex, high-stakes journey, demanding rigorous research, meticulous data analysis, and innovative problem-solving. However, one major hurdle often slows down the process—access to critical, specific, and actionable information. As the pharmaceutical industry grapples with massive volumes of unstructured data, AI-powered search has emerged as a powerful tool that can streamline research and drive discoveries faster. This blog explores how AI-powered search solutions can revolutionize drug development, enhancing efficiency, precision, and insight throughout the process.
The Growing Complexity of Drug Development Data
Drug development has always involved navigating vast amounts of information. However, as research advances, data sources are multiplying—from scientific literature (PubMed, Medline, Embase) and clinical trials to patent documents (FDA, Pat-INFORMED) , health records (EHR) , and real-time data from wearables. The sheer volume and diversity of data are overwhelming traditional search tools, creating significant bottlenecks.
Challenges with Data in Drug Development
- Data Volume: Researchers and scientists have access to an enormous amount of data, but sifting through it can be a full-time job.
- Data Diversity: Drug development relies on both structured and unstructured data, making it challenging to connect insights across these formats.
- Data Silos: Information is often fragmented across departments or stored in inaccessible silos, complicating collaboration and slowing down innovation.
AI-powered search addresses these challenges by breaking down data silos, organizing information for better accessibility, and delivering relevant insights on demand.
Understanding AI-Powered Search
AI-powered search goes beyond traditional keyword-based search by using artificial intelligence, machine learning, and natural language processing (NLP) to analyze and interpret content. This technology can comprehend the context of search queries, identify relationships between concepts, and offer highly relevant results in seconds.
Key Features of AI-Powered Search
- Natural Language Processing (NLP): Understands queries in plain language, so researchers can find answers without complex search strings.
- Machine Learning: Learns from past searches and user behavior to deliver increasingly relevant results over time.
- Entity Recognition: Identifies key terms, such as drug names or disease states, and their connections.
- Predictive Insights: Anticipates researchers’ needs by suggesting related topics or studies.
These features enable AI-powered search to function as an intelligent assistant, capable of responding to complex questions with precise, contextually accurate answers.
How AI-Powered Search Transforms Drug Development Stages
AI-powered search can improve efficiency across various stages of drug development. Here’s a breakdown of its impact:
Target Identification
AI-powered search allows researchers to quickly gather insights on genes, proteins, or pathways associated with specific diseases. It can identify patterns in past studies, uncovering potential drug targets that might otherwise remain hidden.
Preclinical Studies
During preclinical studies, researchers need to access a vast amount of data to understand a compound’s safety and efficacy. AI-powered search enables researchers to access data from previous studies, accelerating the identification of the most promising candidates.
Clinical Trials
AI-powered search optimizes clinical trial design by uncovering insights from previous trials, real-world evidence, and patient data. This helps with participant selection, dosage recommendations, and even predicting potential side effects, ultimately increasing the success rates of trials.
Regulatory Approval
Regulatory requirements are complex, with strict documentation standards. AI-powered search helps teams organize data for regulatory submissions, ensuring that all required information is included and easy to access.
Post-Market Surveillance
After a drug reaches the market, monitoring its real-world impact is essential. AI-powered search can analyze real-time data from medical reports, social media, and other sources, helping pharmaceutical companies track side effects, adapt to emerging risks, and maintain compliance.
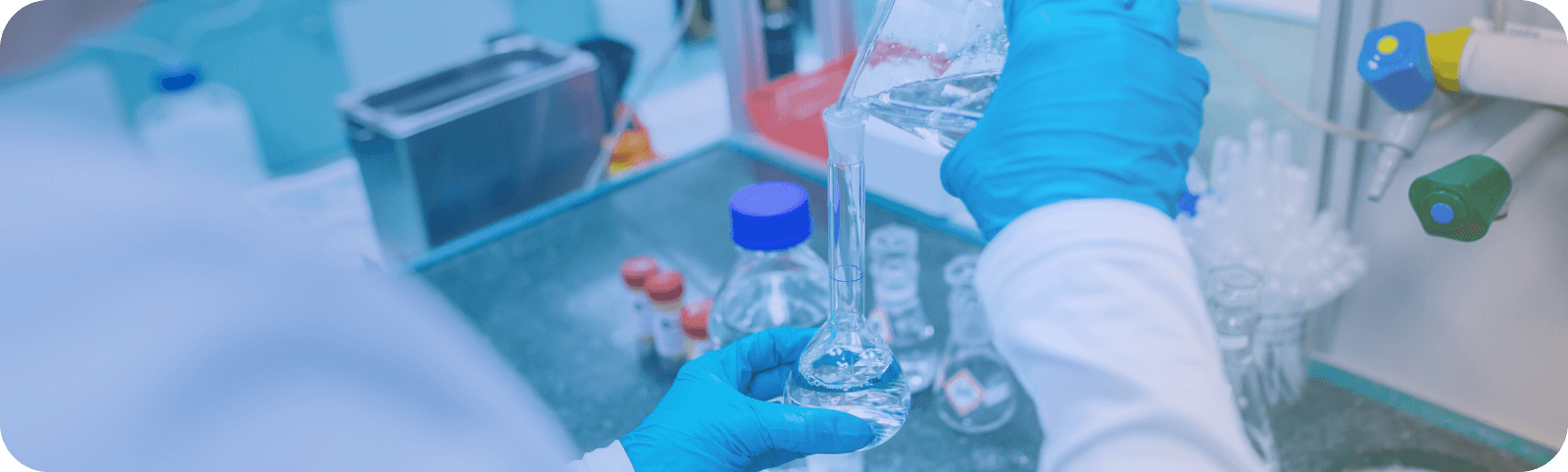
Benefits of AI-Powered Search in Drug Development
Integrating AI-powered search tools into the drug development process offers numerous advantages:
- Accelerated Research: By delivering precise answers instantly, AI-powered search cuts down the time spent sifting through documents and databases.
- Reduced Costs: Faster research and shorter time-to-market translate into significant cost savings.
- Enhanced Collaboration: AI-powered search breaks down data silos, making it easier for teams to access and share insights.
- Improved Decision-Making: AI-powered search can reveal trends and connections that might not be immediately obvious, supporting evidence-based decisions.
- Higher Success Rates: With better information, teams can make more informed choices throughout the process, increasing the likelihood of successful outcomes.
Real-World Use Cases and Future Opportunities
AI-powered search is already reshaping the pharmaceutical and life sciences industries, enabling researchers and scientists to work more effectively. Sinequa has been at the forefront of this transformation, with multiple success stories demonstrating the powerful impact of AI-driven search in drug development.
Sinequa Success Stories in Life Sciences
- Accelerating Research and Development: One global pharmaceutical company used Sinequa’s AI-powered search to streamline access to scientific literature, clinical trial data, and other vital information sources. By implementing Sinequa, the company significantly reduced time spent on data retrieval, enabling researchers to quickly analyze information from thousands of documents and identify potential drug candidates more efficiently. This faster access to insights supported a more agile approach to R&D, bringing promising drugs closer to market.
- Enhancing Knowledge Sharing and Collaboration: Sinequa’s search solution helped another life sciences organization break down data silos, integrating knowledge from different departments, databases, and formats. By making critical research accessible across teams, Sinequa enabled seamless collaboration among scientists and clinicians. This increased information-sharing capacity led to improved decision-making throughout the drug development cycle, empowering teams with the insights they needed to address complex challenges collaboratively.
- Optimizing Clinical Trial Design: For a company conducting multiple clinical trials, Sinequa’s AI-powered search was instrumental in improving trial design and execution. By surfacing insights from previous trials, patient data, and real-world evidence, Sinequa helped the organization better match patients to trials, optimize trial protocols, and predict potential outcomes. This led to enhanced efficiency in the clinical trial process, reducing costs and increasing the likelihood of successful results.
For more details on how Sinequa’s solutions have transformed other life sciences companies, explore our customer stories in life sciences.
Future Opportunities
As AI-powered search technology continues to evolve, the potential applications for drug development will only expand. Future advancements, such as generative AI and machine learning, promise to make AI-driven insights even more powerful. For instance, generative AI could suggest innovative research approaches, predict compound interactions, or identify previously unexplored relationships, further accelerating the drug discovery process.
In an era where innovation and speed are essential, leveraging AI-powered search like Sinequa’s offers life sciences companies a robust, future-proof solution. Embracing these advanced tools will enable organizations to drive more meaningful, faster, and cost-effective research outcomes, ultimately bringing new treatments to patients more efficiently.
Conclusion
The complexity and competitive pressures of drug development make it crucial for pharmaceutical companies to adopt tools that enhance research capabilities. AI-powered search provides a significant advantage, enabling faster, smarter, and more accurate research across the drug development lifecycle. By empowering researchers to access critical information when they need it most, AI-powered search is transforming drug development from a laborious, time-consuming process into a more efficient and effective one.
For companies invested in innovative solutions, AI-powered search represents not just a tool, but a strategic asset. Embracing this technology could be the missing piece to unlocking faster, safer, and more cost-effective drug discovery and development.